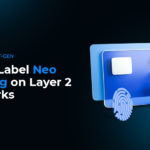
A Comprehensive Guide On White Label Neo Bank Development on Layer2 Blockchain Network
December 3, 2024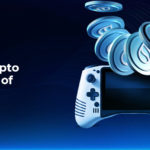
Top Sui Games of 2025 and How to Build One
December 4, 2024In today’s fast-paced world, ‘digitalization’ has become one of the trendy terms that is reshaping the very fabric of industries and economies. This digital revolution requires a massive amount of data to flow every second between devices and sensors. The speed at which this data flows might impress you, however, identifying unusual patterns or anomalies can be challenging. Whether it’s detecting fraud in financial transactions, predicting machine failures, or identifying irregular network activities, they all can cause a huge financial loss to organizations. Anomaly detection is indispensable to optimize operations, mitigate risks, and ensure security.
Anomaly detection using generative AI would be a smart move in the data-driven environment. From healthcare and finance to entertainment, generative AI anomaly detection, with its ability to model complex systems and uncover hidden patterns, is revolutionizing how anomalies are detected and addressed. This blog delves into the transformative role of Generative AI in anomaly detection, highlighting its benefits and use cases—highlighting reasons to consider partnering with a reputable company offering generative AI development services.
Understanding Generative AI in Anomaly Detection
Anomaly detection is a process of identifying unusual patterns or behaviors in data that do not match the expected or normal behavior and signals potential issues, such as fraud, system failures, or cybersecurity threats. It’s like finding a “red flag” that something might be wrong or different from what is typically seen. Traditional methods basically rely on predefined rules or statistical models, however, they often struggle with complex or evolving datasets.
For example, imagine a bank noticing a sudden large withdrawal from an account that’s usually inactive. This would be considered an anomaly, and the bank may want to investigate further.
The Role of Generative AI in Anamoly Detection
Generative AI can help with anomaly detection in various ways. The technology utilizes advanced algorithms that help learn from vast amounts of data and simulate normal system behavior. Anomaly detection using generative AI can understand typical data patterns and pinpoint irregularities (like a sudden change in patterns or unexpected behavior) with exceptional precision. It can even predict these anomalies before they happen which enables organizations to take preventive measures early to avoid problems like equipment failure or security breaches.
Why Choose Generative AI for Anomaly Detection?
The following are the ways showing generative AI anomaly detection can be beneficial for your business-
-
Superior Ability to Handle Complex Data
Generative AI is popular for high-dimensional, non-linear datasets where traditional algorithms struggle a lot. The technology can understand intricate patterns, which enables effective anomaly detection in environments with complex data structures, including time-series data, images, or multivariate sensor readings.
-
Dynamic Learning for Evolving Systems
Another advantage of choosing generative AI anomaly detection is its dynamic learning nature. Unlike static rule-based systems, Generative AI models can adapt to evolving patterns in real-time, which is essential for industries, such as cybersecurity and predictive maintenance, where anomalies often emerge from changing system behaviors.
- Enhanced Precision with Context Awareness
Generative AI can learn the context of normal data, which helps minimize false positives and negatives. This contextual understanding is imperative, especially for use cases, such as healthcare and finance, where accuracy directly impacts outcomes.
-
Scalability for Large Datasets
Generative AI is considered perfect for big data environments as it can process massive, multidimensional datasets. For industries generating terabytes of data, such as IoT, e-commerce, and telecommunications, generative AI anomaly detection is a game-changer.
-
Versatility Across Domains
From fraud detection in financial transactions to anomaly detection in medical imaging, Generative AI’s versatility enables its application across diverse sectors. Its adaptability ensures that it can meet the unique challenges of each domain.
-
Proactive Anomaly Prediction
Generative AI doesn’t just detect anomalies but can also predict them before they occur. This capability is crucial for preventive measures in predictive maintenance and risk management. This quick action helps organizations address potential issues before they escalate into major problems.
-
Efficient Real-Time Detection
Generative AI-powered systems can analyze and flag anomalies in real-time which helps in swift responses. This is crucial for applications like network security and fraud prevention, where delays can lead to significant consequences.
-
Integration with Advanced Technologies
Generative AI can be seamlessly integrated with other technologies, such as edge computing, federated learning, and Explainable AI (XAI), to boost detection capabilities while maintaining data privacy and transparency.
-
Improved Accuracy in Identifying Anomalies
Generative AI models also understand the underlying distribution of normal data, which allows them to detect even subtle deviations. This results in highly accurate anomaly detection, which minimizes errors and improves reliability.
-
Cost Efficiency Over Time
While the initial implementation may require investment, Generative AI anomaly detection reduces costs in the long term by minimizing downtime, preventing failures, and automating anomaly detection processes.
Use Cases of Generative AI in Anomaly Detection
-
Financial Fraud Detection
Challenge: The financial sector handles millions of transactions daily, and spotting fraudulent activity among them can be challenging. Fraudulent transactions often share similarities with normal ones, which makes detection difficult without advanced methods.
Solution: The use of Generative AI for anomaly detection is a wise move as this approach can help model the normal flow of financial transactions and detect subtle anomalies. It flags unusual transactions that deviate from the learned pattern, such as odd spending behaviors, out-of-place transaction amounts, or unauthorized access from unfamiliar locations. By analyzing transaction data over time, the model can identify fraud at an early stage, which reduces losses and fuels the financial security systems.
-
Healthcare Diagnostics
Challenge: In the healthcare sector, the accurate and early detection of diseases is crucial. Medical data, including images (like X-rays or MRIs) and patient records, can contain complex patterns that traditional detection methods struggle to identify.
Solution: Using generative AI for anomaly detection helps analyze vast amounts of medical data, learning what constitutes normal health patterns. It can detect abnormalities in medical scans or patient data that might indicate the onset of diseases, such as cancer, heart conditions, or rare genetic disorders. The system flags outliers that would be missed by human doctors or simpler AI models. Generative AI anomaly detection can help in early diagnosis and better treatment planning.
-
Predictive Maintenance in Industries
Challenge: Unplanned equipment breakdowns in industries can lead to expensive repairs and production downtime. Identifying wear and tear before it results in failure is critical, but traditional systems may miss early signs of equipment failure.
Solution: Generative AI monitors the data from sensors installed on industrial machinery that can identify patterns of normal machine performance. It flags any anomalies that indicate the need for maintenance, such as unexpected vibrations, temperature fluctuations, or irregular operational metrics. This proactive approach of using generative AI for anomaly detection addresses issues before they escalate and helps reduce downtime, improve operational efficiency, and avoid costly repairs.
-
Cybersecurity
Challenge: Cybersecurity threats, including advanced persistent threats (APTs) and zero-day attacks, often evolve at a rapid pace. The conventional security system can struggle to detect new, unknown attack methods or unusual network behavior.
Solution: Generative AI anomaly detection learns the typical behavior of network traffic and user activity. It then identifies deviations, such as unauthorized access attempts, abnormal data transfers, or suspicious login times. Since it can adapt to new patterns, this approach provides an additional layer of security against emerging threats, which helps detect novel cyberattacks that do not follow known attack signatures.
-
Retail and E-commerce
Challenge: E-commerce platforms deal with vast amounts of user data, including shopping behaviors, inventory data, and user reviews. Detecting fraudulent activities, like fake reviews or unusual purchasing patterns, can be difficult with traditional methods.
Solution: Generative AI can analyze purchasing and review patterns to identify outliers, such as sudden spikes in product reviews or purchasing activity that are inconsistent with normal behavior. It can also detect anomalies in pricing, inventory management, or user behavior, which helps retailers maintain the integrity of their platforms and prevent fraud. Additionally, this enables personalized product recommendations by identifying what constitutes “normal” shopping behavior for individual users.
-
Environmental Monitoring
Challenge: Detecting anomalies in environmental data (such as air quality, water levels, and climate patterns) can be difficult, as the data is often noisy and can fluctuate naturally over time.
Solution: Generative AI anomaly detection can model the normal behavior of environmental systems, such as weather patterns or pollutant levels. It learns from historical data and can detect unusual fluctuations that could indicate the onset of natural disasters, pollution spikes, or climate changes. For example, it can flag abnormal temperature rises or sudden shifts in air quality, enabling early warnings for environmental hazards like wildfires, hurricanes, or floods.
Conclusion
Generative AI anomaly detection has emerged as an innovative approach that industries can leverage to uncover hidden patterns in complex systems. Its ability to learn and adapt to data makes it indispensable for applications ranging from fraud detection to healthcare diagnostics. However, as with any technology, expert technical assistance is a must and in this journey, we at Antier can help you.
Antier is a renowned generative AI development company that has sheer expertise in harnessing the potential of generative AI for anomaly detection and driving innovation and efficiency across industries. Backed by 700+ AI experts, we engineer state-of-the-art solutions that help organizations transition from reactive problem-solving to proactive decision-making in the increasingly complex data-driven world. Get in touch with us to get started with our services.