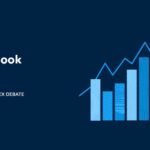
Deciphering Perpetual DEX Development: Order Book vs. AMM
September 6, 2024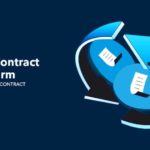
Smart Contract Attacks and Protections: The Importance of a Smart Contract Audit
September 9, 2024Generative AI, a subfield of artificial intelligence that focuses on creating new content, has revolutionized many sectors over the last few months and the pharmaceutical industry is one of them. As the demand for more cost-effective drug solutions grows, leveraging advanced generative AI development services has become essential to stay ahead in the market. This blog explores the challenges in traditional drug discovery and the transformative role of Generative AI in drug discovery process.
Challenges in Traditional Drug Discovery Process
The conventional drug discovery process is time-consuming & costly and requires years of research & billions of dollars to bring a new drug to market. The complexity involved in identifying potential drug candidates, testing for efficacy and safety, and undergoing multiple clinical trial phases creates a bottleneck in the system. Some of the most pressing challenges in traditional drug discovery include:
High Costs
The costs associated with drug discovery and development are substantial, often running into billions of dollars. This includes expenses related to research, preclinical and clinical testing, and regulatory approval. The high cost is driven by the need to test millions of compounds to find one potential drug candidate. On average, it takes between $314 million to $4.46 billion to bring a new drug to market, a financial burden that many pharmaceutical companies struggle to bear.
Time Consumption
On average, the traditional drug discovery process takes 10-12 years to complete. This extended timeline results from the various stages of development, from early discovery and preclinical research to clinical trials and regulatory approval. Each of these stages is lengthy due to manual testing, lengthy data analysis, and regulatory requirements. Time delays are also caused by setbacks during clinical trials, where many drug candidates fail to meet safety or efficacy standards.
Low Sucess Rates
The success rate for drugs entering clinical trials is alarmingly low, with only about 12% of drug candidates receiving regulatory approval. This high failure rate is primarily due to unforeseen issues such as toxicity, lack of efficacy, or adverse reactions in human subjects. Such failures typically happen in the later stages of development, after substantial investments of time and resources, which leads to significant financial setbacks for companies.
Limited Chemical Diversity
Traditional drug discovery methods rely heavily on trial and error, which often limits the ability to discover novel molecular structures. The process of designing new molecules is labor-intensive and constrained by existing knowledge and data. As a result, many drugs are developed based on known chemical entities, which can limit innovation and hinder the discovery of breakthrough therapies for complex diseases.
Regulatory Hurdles
Navigating regulatory requirements is one of the most time-consuming aspects of drug development. Ensuring compliance with strict regulations for safety, efficacy, and quality can delay drug approval and increase the overall cost of development. Any missteps in the regulatory process can result in costly delays or rejections.
Given these hurdles, the application of Generative AI in drug discovery could turn out to be a game changer and enable pharmaceutical companies to streamline and optimize various stages of the development process.
Role of Generative AI in Drug Discovery Process: A Step-by-Step Procedure
Step 1: Target Identification and Validation
Identifying the correct biological target, usually a protein or gene, responsible for a disease is a critical first step in drug discovery. Generative AI in drug discovery accelerates this phase by analyzing massive biological datasets, including genomic, proteomic, and phenotypic information.
Using advanced machine learning models, AI can predict which proteins or genes are likely to be key drivers of a disease. Furthermore, AI algorithms simulate the interactions between drug molecules and biological targets to validate whether a target will respond to treatment.
By predicting the likelihood of a successful drug-target interaction early on, generative AI helps pharmaceutical companies eliminate unsuitable targets quickly.
Step 2: Hit Discovery and Molecular Generation
The discovery of “hits”, chemical compounds that have a high probability of interacting with the identified biological target, is another significant step. Generative AI plays a transformative role in this phase by enabling the creation of entirely new molecular structures. Through deep learning models, drug discovery using generative AI can generate novel compounds with specific properties, such as high affinity for the target, solubility, and low toxicity.
These AI-generated molecules can be designed to match pre-specified chemical and biological parameters. By reducing the need for physical screening and leveraging computational simulations, generative AI in drug discovery accelerates the identification of promising drug candidates.
Step 3: Lead Optimization
Once a hit compound has been identified, it must be optimized to improve its efficacy, safety, and pharmacokinetics. GenAI in drug development automates and accelerates lead optimization by predicting how modifications to a molecule will affect its biological activity and safety. AI models can evaluate various molecular configurations and predict their interactions with biological targets, as well as their toxicity profiles.
Additionally, Generative AI can identify structural changes that enhance the drug’s bioavailability and stability. This rapid iteration allows pharmaceutical companies to optimize drug candidates with unprecedented speed and precision.
Step 4: Preclinical Testing
Generative AI models enhance preclinical testing by predicting how a drug candidate will perform in biological systems. By leveraging large datasets of preclinical data, AI can simulate potential toxicity, efficacy, and pharmacokinetics before any physical testing occurs. This early prediction allows companies to eliminate drug candidates that are likely to fail.
Moreover, drug discovery using generative AI improves the safety profile of drug candidates by identifying potential off-target effects or adverse reactions earlier in the process. By providing more accurate predictions, AI enables companies to move only the most promising candidates to the next phase.
Step 5: Clinical Trials
Clinical trials are one of the most critical and expensive stages of drug development. Generative AI has a significant role in optimizing clinical trial design and execution. By analyzing real-world data, patient demographics, and genetic information, AI can predict which patients are most likely to respond favorably to a new drug. This precision allows for better patient stratification, ensuring that trials are more efficient and have a higher success rate.
Additionally, genAI in drug development assists in the identification of biomarkers that can predict patient response to a treatment, enabling more targeted therapies. By monitoring trial data in real-time, AI can detect early signs of efficacy or safety concerns and allow for adaptive trial designs that minimize risks and improve outcomes.
Step 6: Regulatory Submission and Approval
Generative AI can streamline the regulatory submission process by automating the generation of reports and analyzing past regulatory submissions to identify potential areas of concern. AI can predict how a regulatory body may respond to certain data points and enable companies to preemptively address issues and reduce the likelihood of delays.
Moreover, generative AI in drug discovery provides robust predictive models that simulate long-term safety and efficacy outcomes, which can assist regulatory agencies in making faster, more informed decisions. This enhances the probability of quicker approval and reduces time-to-market for new drugs.
Step 7: Post-Market Surveillance
Once a drug has been approved and brought to market, companies must continue to monitor its safety and efficacy in the real world. Generative AI enables proactive post-market surveillance by continuously analyzing real-world data from electronic health records, social media, and patient reports.
AI models can detect early signs of adverse events, flagging them for further investigation. This allows companies to take corrective actions such as revising dosage instructions or issuing warnings before widespread issues arise.
Conclusion
The integration of Generative AI in drug discovery is revolutionizing the pharmaceutical industry by reducing the time, cost, and risk associated with traditional drug development. From molecule generation to clinical trial optimization, AI-driven solutions are accelerating the pathway to bringing new therapies to market.
For businesses looking to stay competitive in this rapidly evolving field, partnering with a generative AI development company like Antier offers the expertise, scalability, and cost efficiency needed to implement AI solutions effectively. As the role of GenAI in drug development continues to expand, those who embrace this technology will be well-positioned to lead the next wave of innovation in healthcare.