
How GameFi is Revolutionizing the Future of Play-to-Earn (P2E) Models in 2024
September 11, 2024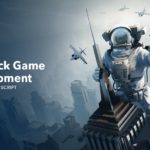
Web3 Game Script – Proven Strategy For Fast Track Game Development
September 12, 2024The pharmaceutical industry has long faced the challenge of discovering and developing new drugs. While a report predicts that the estimated cost for developing a new drug ranges from $314 million to $4.46 billion, another study conveys that traditional drug discovery process takes almost 10-12 years to bring a fresh drug to the market.
The integration of generative AI into drug discovery is transforming the landscape of pharma industry these days. Generative AI in drug discovery allows pharmaceutical companies to optimize workflows, predict molecular behaviors, and design new compounds with unprecedented accuracy and efficiency.
With its ability to analyze vast datasets, predict outcomes, and generate new hypotheses, GenAI is reshaping the future of drug development. In this blog, we’ll explore the top 10 use cases of drug discovery using generative AI and the primary benefits of partnering with a generative AI development company.
Top 10 Use Cases of Generative AI in Drug Discovery
1. De Novo Drug Design
De novo drug design, or the creation of entirely new molecules from scratch, is one of the most transformative applications of generative AI in drug discovery. Traditionally, drug design relied on modifying existing molecules, which limited the exploration of novel chemical spaces. GenAI in drug development uses deep learning algorithms, such as GANs(Generative Adversarial Networks) and VAEs(Variational Autoencoders), to create novel molecular structures that meet specific therapeutic needs.
Generative AI models can design compounds that maximize potency, minimize toxicity, and target specific biological systems. By automating this process, GenAI accelerates the discovery of new drug candidates and opens the door to treating diseases that were previously considered untreatable.
2. Molecule Design
Molecule design is a crucial step in drug discovery, where researchers aim to design chemical compounds that interact effectively with biological targets. Traditionally, this process involved trial and error which requires extensive time and resources. Generative AI in drug discovery speeds up this process by automating the generation of novel molecular structures based on desired properties such as efficacy, stability, and solubility.
AI models can quickly explore the chemical space and offer suggestions for new molecules that meet the required specifications. This has the potential to drastically reduce the time it takes to identify viable drug candidates which gives pharmaceutical companies a significant competitive edge.
3. ADMET Predictions
ADMET (Absorption, Distribution, Metabolism, Excretion, and Toxicity) properties are crucial for determining whether a drug candidate will be successful in clinical trials. Poor ADMET properties are one of the leading causes of failure in drug development. Drug discovery using generative AI offers advanced predictive capabilities by analyzing massive datasets to forecast how a molecule will behave in the human body.
By predicting ADMET properties early in the drug development process, generative AI models help researchers eliminate potentially harmful or ineffective drug candidates before they reach costly clinical trials. This can save companies significant resources and improve the success rate of drug development programs.
4. Drug Repurposing
Drug repurposing involves identifying new therapeutic uses for existing drugs. Generative AI in drug discovery enables faster and more effective drug repurposing by analyzing large datasets of known compounds and predicting their potential applications in treating different diseases. GenAI models can recognize molecular similarities and patterns that suggest alternative therapeutic uses.
This approach has been particularly effective in areas such as oncology, infectious diseases, and neurodegenerative conditions. Drug repurposing is a cost-effective strategy that reduces the risk and expense associated with developing entirely new drugs, while still offering the potential for breakthrough treatments.
5. Protein-Ligand Interaction Prediction
Understanding how a drug molecule interacts with its biological target is essential in drug discovery. Protein-ligand interaction prediction helps determine whether a drug candidate will effectively bind to its target and produce the desired therapeutic effect. Generative AI in drug development leverages deep learning models to predict these interactions based on the chemical structures of both the ligand and the protein.
GenAI models can simulate and predict how different molecules will bind to target proteins while helping researchers focus on drug candidates with the highest potential for success. By improving the accuracy of protein-ligand interaction predictions, pharmaceutical companies can reduce the number of failed drug candidates and speed up the development timeline.
6. Virtual Screening
Virtual screening is the process of using computational methods to evaluate large libraries of chemical compounds and identify potential drug candidates. Drug discovery using generative AI takes virtual screening to the next level by efficiently processing vast amounts of data and predicting which compounds are most likely to exhibit desired biological activities.
AI models can rapidly narrow down vast compound libraries to a few promising candidates, significantly reducing the time and cost required for experimental screening. This process is especially beneficial in early-stage drug discovery when researchers need to identify lead compounds for further development.
7. Toxicity Prediction
One of the most critical challenges in drug development is ensuring that new compounds are safe for human use. Toxicity prediction involves forecasting how toxic a drug candidate will be to the human body. Generative AI in drug discovery has proven highly effective in predicting toxicity levels based on chemical structure and biological activity data.
By identifying potential toxicity issues early in the drug development process, generative AI reduces the likelihood of late-stage failures in clinical trials. This not only saves pharmaceutical companies substantial resources but also helps protect patients from harmful side effects.
8. Antibody Design
Antibodies are essential components in developing therapies for diseases such as cancer, autoimmune disorders, and infectious diseases. Designing antibodies that can specifically target pathogens or cancer cells is a complex task. GenAI in drug development assists by generating novel antibody structures optimized for binding affinity and specificity.
By using generative AI, pharmaceutical companies can design antibodies that are more effective at targeting disease-causing molecules while minimizing unwanted side effects. This technology accelerates the discovery of new biological drugs and immunotherapies, offering new hope for treating a range of diseases.
9. Compound Library Expansion
Pharmaceutical companies maintain vast libraries of chemical compounds that can be screened for potential drug candidates. However, these libraries are often limited in scope. Generative AI development companies are using AI models to expand compound libraries by generating new chemical structures that meet specific criteria.
By augmenting existing libraries with AI-generated compounds, researchers can explore a broader chemical space and increase the likelihood of discovering novel drug candidates. This approach not only speeds up the drug discovery process but also enhances innovation by introducing entirely new molecular structures into the pipeline.
10. Automated Synthesis Planning
Once a promising drug candidate is identified, the next challenge is figuring out how to synthesize the molecule in a laboratory. This process, known as synthesis planning, can be highly complex and time-consuming. Drug discovery using generative AI streamlines synthesis planning by automating the process of generating step-by-step chemical synthesis routes.
AI models can analyze chemical databases and design optimal synthesis pathways for new compounds. This reduces the time and cost associated with bringing a new drug candidate from concept to production, further enhancing the efficiency of the drug development pipeline.
The Role of Generative AI Development Companies
While the potential of Generative AI in drug discovery is immense, the complexity of implementing and maintaining AI models requires specialized expertise. Partnering with a Generative AI development company allows pharmaceutical firms to harness the power of AI without the need to build in-house teams from scratch. Here are some key advantages of partnering with a generative AI development company:
- Expertise in AI and Drug Discovery
A reputable generative AI development company brings together domain experts in both AI and drug discovery. This ensures that the AI models developed are tailored to the specific challenges of pharmaceutical R&D.
- Cost and Time Efficiency
By outsourcing AI development, pharmaceutical companies can reduce the costs associated with hiring, training, and maintaining in-house AI teams. This also allows for quicker implementation, as AI development firms are already equipped with the tools and expertise needed to hit the ground running.
- Scalability
As the demand for AI-driven drug discovery grows, partnering with a Generative AI development company ensures that your organization can scale its AI capabilities without overhauling its existing infrastructure. AI development companies can provide scalable solutions that grow with your business needs.
- Compliance and Data Security
Working with a trusted AI partner ensures that compliance with regulatory standards and data security protocols is maintained throughout the drug development process. This is particularly important given the sensitive nature of pharmaceutical research and patient data.
Conclusion
From de novo drug design to ADMET predictions and antibody design, generative AI is driving new breakthroughs and significantly reducing the time and cost associated with developing new drugs. For pharmaceutical companies looking to stay ahead in an increasingly competitive landscape, partnering with a generative AI development company like Antier can unlock the full potential of AI-driven drug discovery.